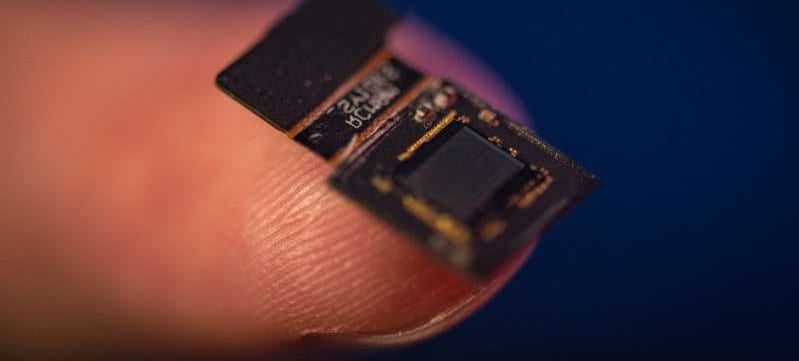
Rice Computational Imaging Lab
Our lab focuses on solving hard and challenging problems in imaging and vision by co-designing sensors, optics, electronics, signal processing, and machine learning algorithms. This emerging area of research is called computational imaging or more generally computational sensing. Our group is generally application-agnostic and focuses on developing foundational theories, tools, techniques, and systems.
Research
|
Publications
|
Datasets
|
Team |
Code |